Overview
GERMS is a dataset for active object recognition, consisting of recordings of 1365 give-and-take trials between different people and a humanoid robot. In each give and take trials, an object is handed to the robot, that receives the object and actively examines it. For each trial, this information is stored: frames from the robot's camera and the position of the robot’s servo motors. The statistics of the dataset is shown in table below.
Collection | Number of tracks | Frames in each track | Annotated frames in each track |
Train | 816 | 265±7 | 157±12 |
Test | 549 | 265±7 | 145±19 |
A sample give-and-take trial is shown in this video:
Objects
The object set we use for GERMS data collection consists of 136 stuffed toys of different microorganisms. The toys are divided into 7 smaller categories, formed by semantic division of the toy microbes. The motivation for dividing the objects into smaller categories is to provide benchmarks with different degrees of difficulty. Here is a collage of all objects used in GERMS dataset.

Table of Germs (click for additional videos)
Annotations
The annotation for train set is done manually and includes the bounding box of objects in the images. Train set annotations are available along with the dataset. For examples of annotations, see Benchmarks.
Benchmarks
We define 16 benchmarks on the GERMS dataset, 2 for each of the 8 categories of objects. For the first benchmark, methods are allowed to use human-annotated object bounding boxes for both train and test sets. Methods should report the accuracy of label prediction for object in each category vs. the number of observed views. Here is the baseline for the first 8 benchmarks:
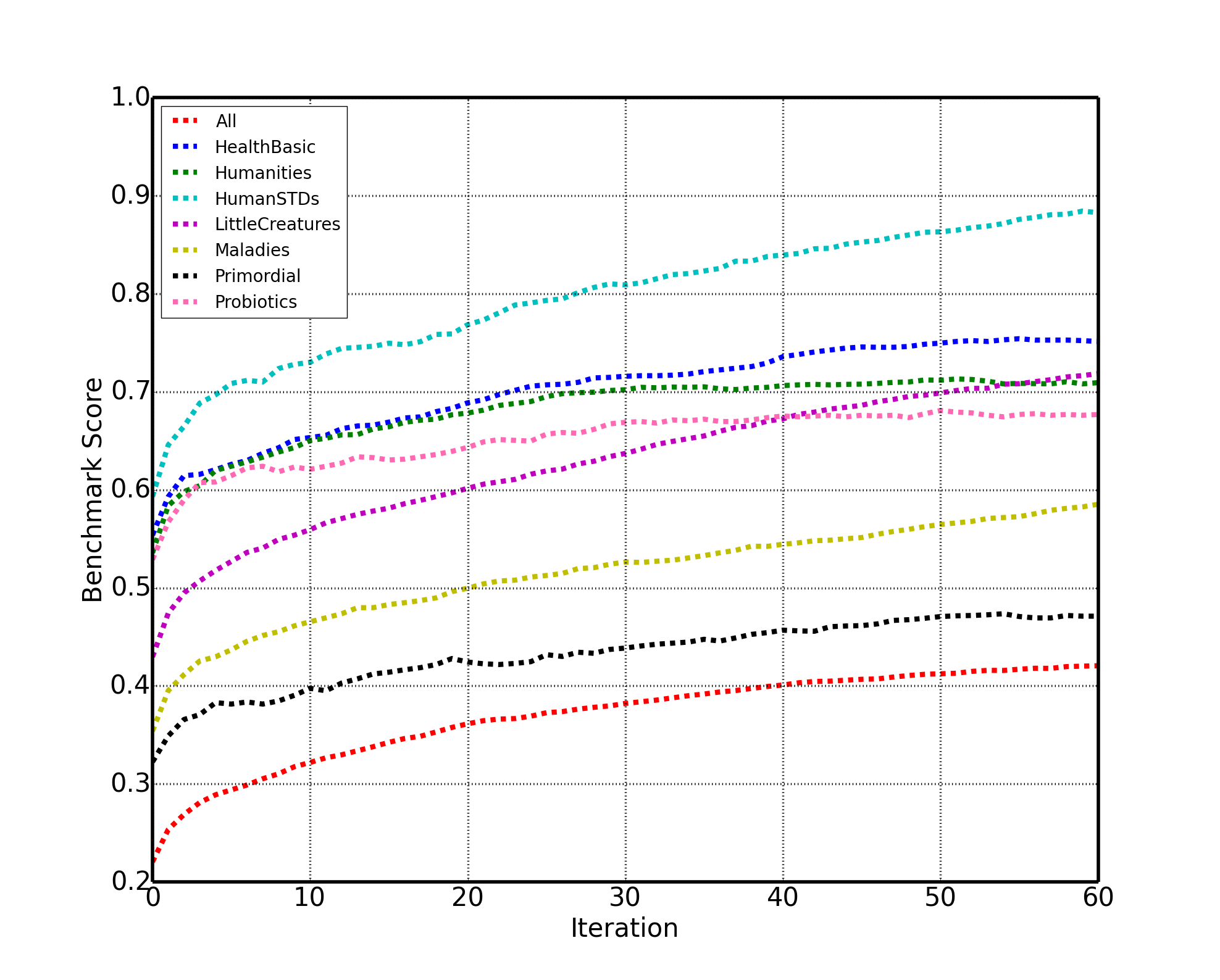
For the second benchmark, one is allowed to use human-annotations only for train data. These benchmarks are more challenging since the backgroud is non-simple. Here are the baseline results for these benchmarks:
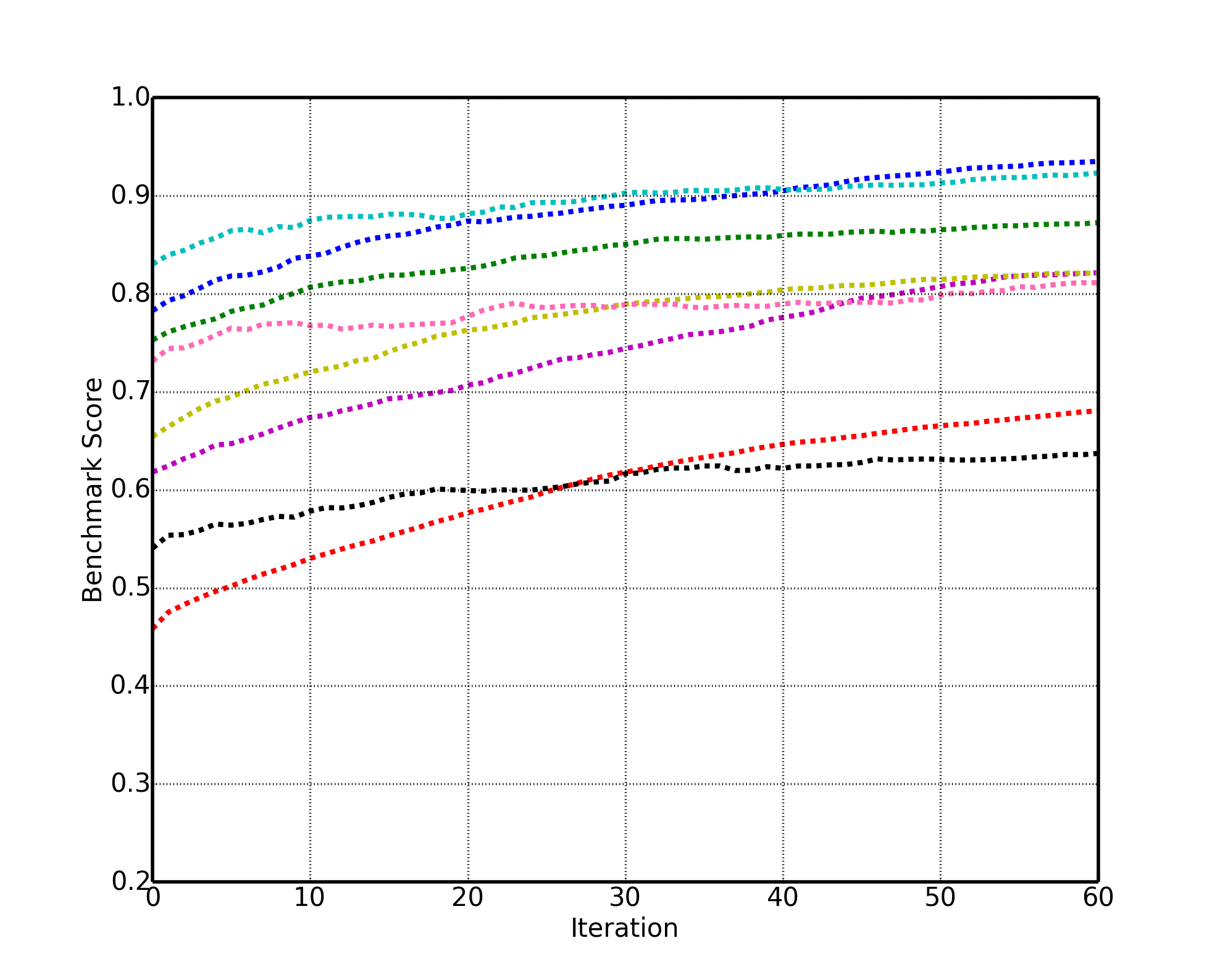
Here is a video of active object recognition. The left image is the original video stream, the middle image is the human annotated and the right image is the result of automatic annotation. Above each image, a histogram shows the degree of belief of the identity of the object in image. The correct object is shown with purple background.
How to obtain
The size of the dataset is more than 2.5 TB. If you are interested in obtaining the dataset, please send an email to: mmalmir@eng.ucsd.edu.
Cite GERMS
Please cite the dataset using the following:
Malmir M, Sikka K, Forster D, Movellan JR, Cottrell G. Deep Q-learning for Active Recognition of GERMS: Baseline performance on a standardized dataset for active learning. InBMVC 2015 (pp. 161-1). pdf
Acknowledgement
This work was supported by NSF grant IIS-0808767.